INTRODUCTION
FROM auditory studies of "thin slices" of music, we know that people can hear a great deal in very brief snippets of sound. For instance, emotional qualities such as "happy" or "sad" may be reliably discriminated even based on half a second of music (Peretz et al., 1998; see also Bigand et al., 2005), and listeners' liking judgments of music can already be accurate after a 750-ms auditory exposure (Belfi et al., 2018). Genre judgments, too, can be accomplished surprisingly well after hearing less than a second of musical sound. Gjerdingen and Perrott (2008) demonstrated that non-musicians' choice of genre labels for 250-ms sound clips agreed in 44% of the cases with their labeling based on 3-s clips. In a study by Mace and colleagues (2011), participants chose between five genre labels, and performed well above chance at sample lengths between 125 and 1000 ms. In Krumhansl's (2010) study, consistent judgments of style and decade of release were possible on the basis of 300-ms sound clips of popular music; at 400 ms, even artist and song title were recognized in more than 25% of the trials.
The present study was motivated by the question whether similar feats of recognition might be possible based on brief visual exposure to musical notation. In the visual domain, quick recognition takes place with many everyday objects (Grill-Spector & Kanwisher, 2005) and especially in face recognition. Beyond simply recognizing a familiar person, quick face perception may also involve making complex social judgments about aspects such as personality (Willis & Todorov, 2006), emotion (Martinez et al., 2015), or sexual orientation (Rule & Ambady, 2008). This could be compared to the above-mentioned cases of auditory genre recognition in music: in both cases, a quick perceptual exposure triggers complex, culturally constructed concepts that may involve holistic, subjective understandings of qualities and values. Whereas we all are "experts" in face recognition, grasping such more holistic aspects of written music of course requires some specialized skills in music reading. However, with such skills, musicians might similarly be able to recognize the musical style or assess the aesthetic qualities of the music from very brief glances at the notation. Whether this is so will be explored in the present study.
In the musical domain, a crucial difference between auditory and visual brief exposure has to do with the amount of music involved. While one second of listening exposes the listener to exactly one second of music, one second's visual exposure to a musical score could, in principle, expose the viewer to a lot more. A quick glimpse at the score could be used to form a quick understanding of the contents in a longer stretch of music. In music reading research, such quick overviews have not often been addressed, which probably has to do with the usual concern for reading music in the context of playing an instrument. Indeed, research suggests that pianists might not even need to see more than one bar at a time in order to perform flawlessly (Gilman & Underwood, 2003; Truitt et al., 1997). Even for the sight-reading musician, though, quick visual overviews might come in handy for guiding the upcoming performance. McPherson (1994) proposed that competent sight reading relies on
the ability to seek information relevant to an accurate interpretation prior to the commencement of the musical performance. This involves observing the key and time signature of the work together with an ability to scan the music briefly in order to maximize comprehension and to identify possible obstacles (McPherson, 1994, p. 229).
In a later longitudinal study of young instrumental learners, McPherson (2005) showed that participants' sight-reading achievement was indeed better explained by such preparatory strategies than by their accumulated amount of practice. This suggests that some sight-reading success might be attributed to the efficient self-regulation of the performance that is served by an efficient preview of the music at hand.
Pattern recognition and style recognition
Grasping the gist of musical notation in less time than is required for a performance obviously relies on the ability to recognize familiar patterns. The recall of brief visual displays of notation was studied tachistoscopically even before the advent of cognitive psychology, showing effects of what would now be called pattern recognition or perceptual chunking. Ortmann (1934) presented vertical chords (written in staff notation without a clef) for 400 ms, asking advanced music students to write on staff paper what they had seen. An error analysis allowed him to conclude that chord forms corresponding to regular triads or seventh chords (or their inversions) were read as "higher order groupings." In another early tachistoscopic study, Bean (1938) used a presentation time of 187 ms and a piano performance task, interpreting the results of an error analysis with a distinction between "pattern readers" and "part readers." In Bean's interpretation, pattern readers use "the entire group of notes as a cue to recognition," whereas part readers "see only one or two notes of a group and fill in the remainder subjectively" (Bean, 1938, p. 78).
In their classic chess research, de Groot (1965) and Chase & Simon (1973) demonstrated large differences in the ability of players on different skill levels to reproduce briefly exposed chess game positions. In a similar study concerning musical notation, Sloboda (1976) showed that with exposure times of 150 ms or more, musicians could reproduce arrays of noteheads on clefless staves better than non-musicians. Using longer presentation times (4 s), Halpern and Bower (1982) explored the effects of expertise and musical structure on the memory of notated melodies. Parallel to research on auditory recall (Deutsch, 1980), they showed that the well-formedness of melodic structure facilitated participants' ability to write down the melodies. Notably, however, while the expertise effect in chess vanished with random game positions (de Groot, 1965; Chase & Simon, 1973; Reingold et al., 2001), it seems that even randomly structured notated music is better recognized (Waters, Underwood, & Findlay, 1997; Wong et al., 2020) and reproduced (Sloboda, 1976; Halpern & Bower, 1982) by musically experienced individuals than by novices. This indicates that musicians can attribute some musical meaning even to randomly generated sequences of musical symbols—perhaps by using their skills of notational audiation (see Brodsky et al., 2003; Brodsky et al., 2008). Kalakoski (2007) demonstrated that musicians' advantage in recall over non-musicians indeed persisted even in sequential visual presentation of either note heads or written note names, suggesting an advantage gained from pre-learned musical knowledge and skills.
In the above-mentioned studies of quick recognition of musical notation, the focus has been on the accurate memory and reproduction of note symbols. From such a perspective, experts' processing of music notation has also been shown to involve holistic effects, defined as relative inability to selectively attend to part of an object (Wong & Gauthier, 2010). We mention this to point out the difference to how we used the term "holistic" above. In the present study, we are concerned not with the accurate reproduction of notes, but with the visual impression concerning musical style. We understand style as "replications of patterning" (Meyer, 1989) that take place on a more global level in the musical score, carrying cultural meanings beyond the sum of the individual notes. Musical style cannot necessarily be pinned down to any restricted features of notation, as it involves several musical parameters such as melody, rhythm, harmony, texture, dynamics, as well as higher-level patterning in terms of musical gestures, phrases, and so on.
The holistic aspect involved in the recognition of style from musical notation might be compared to chess experts' recognition of chess game positions as meaningful, strategic configurations, or to expert radiologists' rapid viewing of medical images. Studies in medical image perception have shown that experts in that field can rapidly extract diagnostically relevant information from their initial glimpse of the image, finding a lesion in the tissue in just hundreds of milliseconds (Kundel & Nodine, 1975; for a review, see Sheridan & Reingold, 2017). Here, the experts' visual processes are assumed to commence in a "global" (Nodine & Kundel, 1987), "nonselective" (Drew et al., 2013), or "holistic" (Kundel et al., 2007) mode of perception. Such global analysis involves using parafoveal vision (Brams et al., 2019), but it also arguably involves making appropriate judgements regarding the visual display on the basis of higher-order configurations. In the case of musical notation, experts can similarly be expected to see not just note symbols, but rather music, allowing them to apply appropriate stylistic or aesthetic concepts to what they see. The music might be seen as a "chorale," a "lyrical melody," or a "fugato."
Apart from musicians preparing for performance, quickly grasping the qualities of written music can also come in handy for composers, conductors, or even just music teachers browsing through collections of music in search for suitable material for their students. Arguably, the relevant expert skills at play cannot very well be studied by letting participants choose genre labels from among given alternatives. In real life, musicians would more typically have to search for appropriate concepts from their own prior experience, and their quick previews might even result in mutually different preliminary understandings of the same score. It should also be noted that the common-practice classification of style periods is not objectively present in the music: labels such as "Baroque," "Classicism," or "Romanticism" are interpretative products of later musicological work living in our culture, and might also be challenged.
The rationale for the study
In this study, we assumed that an appropriate way to study something akin to quick auditory genre recognition in the visual domain would be to let musicians freely speak of what they see after being exposed to quick glimpses of musical notation. To take a first exploratory step towards understanding this issue, we chose to work with retrospective verbal protocols (see Ericsson & Simon, 1993) and to focus on pianists—an especially interesting group of music readers because of their typical access to the whole notated structure of the music, and thus to much of its aesthetic qualities. Our first research question was an exploratory one:
RQ1: To what extent do professional pianists gain stylistic information from brief glances at written piano music?
Supposing that pianists would be able to recognize musical style periods even from brief visual displays, it should be of further interest to ask how they are able to do it. Following Ericsson and Simon (1993), we assumed that verbalization about a task may reflect the structure of the thought processes involved, and thus we were also interested in studying the relative timing of spoken statements and the sequences of contents in the protocols. This seemed especially appropriate from the point of view of dual-process views of cognition that make a distinction between (1) intuitive processes that are rapid, automatic, and parallel, and (2) reflective ones that are slow, controlled, and serial (see Betsch, 2008; Betsch & Glöckner, 2010; Evans, 2010a; 2010b; Evans & Stanovich, 2013). On the one hand, style recognition might be based on rapid intuitive processes, where the individual quickly provides a response without consciously inferring it from other information. On the other hand, it could also possibly be based on reflective processes relying on working memory—that is, on step-by-step analytical inferences from the combined cues that are available to conscious reflection. These considerations suggest that the differences between the two kinds of cognitive processes might become evident from the latency of the recognition responses and/or from the amount of information evident in the protocols. Thus, we asked:
RQ2: Based on the latency of style attributions and other spoken contents in verbal protocols, is there evidence that correct recognition of musical style takes place through intuitive thought processes?
Inspired by the research on medical image viewing (see above), we hypothesized that experts' style recognition from musical notation would represent more intuitive than reflective processing. If so, style recognition could be expected to take place (1) more rapidly than responses that are not based on recognition, and (2) without as much available music-analytical content as in cases of non-recognition. When intuitive, effortless recognition fails, however, we could expect reflective, inferential processes to intervene (see Evans & Stanovich, 2013). Such processes might take more time than quick, intuitive recognition, and given the potentially scant evidence from the quick visual display, the resulting inferences might also more often lead to incorrect style attributions. Hypothesizing that style recognition takes place in an intuitive manner, we thus expected that incorrect style attributions might take more time than correct ones, and that that verbal protocols preceding incorrect style attributions might show more music-analytical content than is found in cases of correct recognition. The alternative scenario would be that style recognition takes place in a reflective, analytical manner. In this case, correct recognitions (1) should not be faster than incorrect ones, and (2) participants' verbal protocols could be expected to show more music-analytical content prior to correct recognitions than otherwise.
METHOD
Participants
25 pianists, professionally educated in the classical tradition, volunteered in the experiment. Four participants had master's degrees in music, 11 bachelor's degrees, 8 participants were bachelor-level students, and 2 had vocational upper secondary qualification (the lowest level of professional training in music in Finland). The participants' mean age was 29.6 years (SD = 8.8), and their professional careers as pianists and piano teachers ranged between 4 to 27 years (M = 10.5 years).
In the Finnish national system of higher music education, students of classical piano performance are typically encouraged to explore repertoire from stylistic periods ranging from the Baroque, through Classicism and Romanticism, to 20th century Modernism. The curricula do not include a compulsory core repertoire of works. Hence pianists have a liberty of creating their personalized repertoires, and, in our experience, they are actively encouraged to study less well-known composers as well. In their own free written descriptions of their piano repertoire, the participants indicated their familiarity with the above-mentioned stylistic periods. At the same time, they clearly did not limit themselves to solo piano repertoire, but worked on a daily basis on other individual interests such as chamber music, improvisation, popular styles, accompaniment, children's music, or original compositions.
Stimuli
The visual stimuli consisted of nine score extracts from the keyboard works of J. S. Bach, L. v. Beethoven (of the "Classical" period), and F. Chopin, three from each composer (see Table 1). The works were chosen to represent canonical piano literature that classically trained pianists would be likely to be acquainted with, but avoiding the opening bars with the most characteristic features of the pieces. Each of the extracts comprised three consecutive systems of keyboard notation, and they were all digitally scanned from Hänle Verlag's Urtext editions to obtain consistency in the visual outlook of the stimuli. An example is provided in Figure 1 below.
In silent-reading tasks, experienced music readers' average fixation times have been reported to lie around 200–300 ms (Waters et al, 1997; Penttinen et al., 2013). The exposure time of 500 ms was chosen to allow at least two fixations. This would not suffice for a complete search of the score, but would potentially allow for a holistic recognition process, and a saccade to some location of interest (Kundel et al., 2007).
Procedure
The second author (a professional piano teacher) met the participants for individual peer discussions. The participants were seated at an electronic keyboard, with a computer screen serving as a music stand. The idea was to facilitate musician-to-musician discussions where the participants could freely talk about what they saw on the screen and demonstrate the music at will on the keyboard. For each stimulus, the participants were asked to "describe in your own words everything that you had time to perceive in the notated example," and to "give your impression about the musical style" in question. In order to help the facilitator orient in the discussion (so as not to miss which score excerpt had been shown), we chose to use a single presentation order for all participants (see Table 1), and check for possible order effects afterwards (see below). This seemed justified as our main interest was not in effects due to specific stimulus features, but rather more generally in the kinds of "style talk" that would ensue after brief glances at notation.
Excerpt | Key signature | Bars | Number of notes (RH, LH) | Rests | Acci- dentals | Source |
---|---|---|---|---|---|---|
Beethoven: Sonata op. 2 nr. 3, 1st mvt | C major | 179–193 | 168 (96, 72) | 13 | 24 | HN 32, p. 51 |
Chopin: Waltz op. 64 nr. 1 ("Minute" waltz) | D♭ major | 69–87 | 161 (88, 73) | 8 | 13 | HN 131, p. 40 |
Beethoven: Sonata op. 13 ("Pathétique"), 3rd mvt | C minor | 62–73 | 153 (60, 93) | 6 | 12 | HN 32, p. 159 |
Chopin: Fantaisie-Impromptu, op. 66 | C♯ minor | 15–23 | 252 (144, 108) | 0 | 35 | HN 235, p. 40 |
Bach: Prelude BWV 879 | E minor | 5–18 | 138 (78, 60) | 3 | 14 | HN 16, p. 46 |
Bach: Fugue BWV 886 | A♭ major | 6–11 | 172 (95, 77) | 8 | 13 | HN 16, p. 92 |
Chopin: Ballade op. 52 | A♭ major | 131–138 | 249 (154, 95) | 7 | 101 | HN 938, p. 9 |
Bach: Three-part Sinfonia BWV 799 | A minor | 13–30 | 186 (107, 79) | 2 | 9 | HN 64, p. 58 |
Beethoven: Sonata op. 10 nr. 3, 1st mvt | D major | 18–32 | 178 (79, 99) | 23 | 19 | HN 32, p. 124 |
The stimuli were presented on a computer screen, using the software Microsoft PowerPoint. First, the participants were familiarized to the procedure with two test scores (by Clementi and Schumann). Participants controlled the appearance of the stimuli by using a mouse. On the first click of the mouse, a white square appeared on the screen, outlining the area where the following stimulus would appear. On the second click, the stimulus appeared for 500 ms, followed by a blank screen. On the screen, the size of the stimuli (20 cm in width) matched the printed edition. With approximately 60 cm viewing distance, each stimulus horizontally subtended a visual angle of circa 19°. After each stimulus, the facilitator first allowed the participant to respond alone. When it seemed that the participant did not have more to say, the facilitator took part in the discussion, helping the participant with "content-empty" questions (i.e., ones devoid of information about the musical content, such as "What did you see then?"; see Petitmengin, 2006). The discussion parts of the sessions will not be reported in this article. The sessions were recorded with a video camera.
Content Coding
For this article, we chose to analyze only the participants' initial verbal responses after each stimulus, until the first utterance of the experimenter. The median length of these spoken responses was 52 s (M = 58.1, SD = 29.6). The responses were transcribed word for word and subjected to content analysis as follows.
We devised a coding system involving separate codes for recognition and misattribution of Style (i.e., style period), Composer, and Composition. Each of these recognition categories received two possible codes, with suffixes "C" and "I" indicating correct and incorrect responses, respectively. For instance, mentioning "Baroque" for a Bach piece was coded as Style-C, and mentioning "Bach" for a Beethoven piece would be coded as Composer-I. We also initially included the code Performance for recognizably playing some of the musical piece in question on the keyboard. By a process of constant comparison (Glaser & Strauss, 1967), the second author extended the coding scheme to cover also other spoken contents in the responses. The scheme involved content categories concerning (i) pitch organization, (ii) rhythmic and metric organization, (iii) texture, registral aspects, and the role of hands, (iv) articulation, and (v) experiential qualities. Both authors independently coded all 225 responses using these content categories, yielding a total of 1,602 shared or individual code assignments, including 1.5% discrepancies between the two codings (Cohen's κ = 0.98).
A discussion of the coding led to some modifications regarding the third content category, texture. First, statements regarding compositional type (e.g., "it was a fugue"; "it looked like a minuet") which had first been coded as indicating texture, were given a separate category, type. Second, we realized that statements regarding registral range or the role of hands were always combined with content concerning pitch organization (e.g., "there were triads in the right hand"), temporal organization (e.g. "there were eighth-notes in the bass"), or texture (e.g., "a waltz accompaniment in the left hand"). To avoid counterintuitive alternation between codes in these passages, we removed registral aspects and the role of hands from the texture category, leaving them uncoded. Third, the distinction between statements concerning articulation and texture often seemed hard to draw, and thus we subsumed statements concerning articulation into the texture category.
Apart from these changes, we realized that the recognition category Performance was only needed four times (twice for Beethoven's Sonata Pathétique, twice for Chopin's Minute waltz), and hence we combined this category with the recognition category Composition. A preliminary analysis showed that despite the brief presentation time of the visual stimuli, the right composition was identified in a number of trials (Bach: 9.3%, Beethoven: 5.3%, Chopin: 8.0%). However, given that these recognition percentages were relatively low, we further decided to collapse the Composition codes into the category Composer. With these changes, the coding scheme was revised to the final form shown in Table 2 below. According to the coding, participants' responses included a mean of 5.8 code instances (SD = 2.5), and 4.1 unique codes, on average (SD = 1.4).
We demonstrate our procedure by a coding example of one participant's (Nr. 2) response regarding Bach's Sinfonia in A minor (BWV799; see Figure 1). In this case, the participant recognized the composer after first making some observations regarding Texture, Experience, and Time. Most of the content statements are also quite appropriate, apart from the mistake regarding key (perhaps a Bb note in the score had suggested F major to the participant):
Well, it seemed like [Texture] a polyphonic piece. It occurred to me that it could be [Experience] a rather fast piece. [Texture] In the right hand, simultaneously some kind of melody, [Time] quarter or eighth notes, and at the same time in the right hand a sixteenth-note pattern there. And the left hand might have had eighth notes again. It could be something by [Composer-C] Bach. It brings to mind [Texture] a three-voice… what do you call them… are they [Type] Sinfonias or what. [Pitch] The key was perhaps F major, or maybe something with only few signs in the key signature. This could well be [Time] in three time, perhaps. Maybe 3/4 or 3/8. That's what came to mind.
Label | Criterion | |
---|---|---|
Recognition Codes | Style-C | Correct response regarding the style period (Baroque, Classical, Romantic, or equivalent terms) |
Style-I | Incorrect response regarding style period | |
Composer-C | Correct response regarding the composer (Bach, Beethoven, Chopin); includes mentioning the name of the piece in question or playing some of it on the keyboard, or mentioning the right compositional type along with the right composer name (e.g. "Bach invention") | |
Composer-I | Incorrect response regarding composer | |
Content Codes | Pitch | Pitch organization: key signature, harmony, tonality, chromaticism |
Time | Temporal organization: time signature, meter, rhythm, time values | |
Texture | Textural statements regarding density, number of voices, homo-/polyphony, accompaniment patterns, scale patterns, grouping and phrases, articulation, words such as "melody" and "bass" occurring with descriptive adjectives | |
Type | Compositional type: e.g., "fugue," "invention," "gigue," "sonata," "bagatelle," "nocturne," "Baroque dance," "a small piece," etc. | |
Experience | Aesthetic experience, imagined tempo, difficulty of the piece, time period of composition ("old," "18th century," "more modern," etc.) |
Statistical Analysis
The quantitative analyses were carried out by using only the first code instances of each code type that was present in each trial. For the timing analysis, these first instances were given a time index, measuring the time from the flash of the visual stimulus onscreen to the beginning of the utterance in question (rounded to the nearest second). The analyses were carried out in the R statistical environment (R Core Team, 2019).
For a preliminary check regarding possible effects of the presentation order on the responses, we used Spearman correlation as well as repeated measures correlation. The latter, implemented in the R package "rmcorr" (Bakdash & Marusich, 2021), is a correlational method accounting for non-independence among observations (Bakdash & Marusich, 2017). According to Spearman correlation, the total number of participants recognizing the Style or the Composer of an excerpt was not associated with the trial number of the presentation order (Style: ρ = –0.13; Composer: ρ = –0.08; both ps > .1). According to repeated measures correlations, there was also no common within-individual association between the trial number and the latency of correct recognition (Style: rrm = –0.07; Composer: rrm = 0.14; both ps > .1). Hence, on the group level, the presentation order did not affect either the level of recognition or the latency of giving correct answers.
For estimating participants' sensitivity of recognizing the composers Bach, Beethoven, and Chopin, we used Zhang and Mueller's (2005) non-parametric estimate of sensitivity. However, our main analyses concerned the timing of correct and incorrect recognitions and the contents of the responses between correct and incorrect recognitions. For the timing analysis, we used Wilcoxon rank-sum tests for clustered data (Rosner, Glynn, & Lee, 2003), as implemented in the R package "clusrank" (Jiang, Lee, Rosner, & Yan, 2020). This allowed taking into account that each participant provided responses in several trials. For the content analysis, we used paired t-tests of participant means.
RESULTS
Recognition
Our first research question asked to what extent pianists could extract stylistic information from the brief visual exposure. It turned out that pianists did recognize either musical Style and/or the Composer in an average of 4.4 (out of 9) trials per participant (SD = 2.1), corresponding to almost half (48.9%) of trials in the whole participant group. Style-C was recognized in 30.7% of all trials, or in a mean of 2.8 out of nine trials per participant (SD = 1.7). Composer-C was recognized in 28.4% of all trials, or in 2.6 trials per participant (SD = 1.5). Overall, only two participants did not recognize Style in any of the trials, three did not recognize any Composer, and only one did not recognize either of these aspects in any of the trials. Using a median split, we may note that the 14 best participants recognized either Style-C or Composer-C in 5.9 trials (SD = 2.5), on average.
It is difficult to formally estimate participants' recognition sensitivity, because no response alternatives were announced beforehand, and because responses could contain several guesses, sometimes including both a correct and one or more incorrect responses. It might be noted that the observed recognition of Style-C was lower than one could have achieved by mechanically responding, say, "Baroque" in each trial. However, our participants were much more cautious in their response strategies, only giving one or more Style-I responses in 15.1% of the trials and one or more Composer-I responses in 28.0% of them. While some verbal protocols contained up to four suggested composer names, the mean number of composer names mentioned in a protocol was only 0.67 (SD = 0.85), and no less than 47.8% of the composer names mentioned by an individual participant were right answers, on average (SD = 29.2).
We further estimated participants' sensitivity of recognizing the composers Bach, Beethoven, and Chopin by treating all Composer-C responses as "hits" and all inappropriate attributions of these three composer names as "false alarms." It was assumed that providing one wrong Composer response along with the right one would diminish the recognition success to 0.5 of a hit, two accompanying wrong responses would give a 0.33 hit, and so on. For each participant, we then summed up the number of such modified hit scores across the 9 trials, and similarly counted their individual sums of false alarms (the two other composer names incorrectly used). Excluding two participants with no Composer recognition, Zhang and Mueller's (2005) non-parametric estimate of sensitivity yielded the participant mean value A = 0.78 (SD = 0.09) which is above the midpoint between perfect recognition (1) and chance performance (0.5). Hence, the observed recognition sensitivity would count as relatively good even assuming that the participants would have known beforehand which composers to look for—which they in fact did not know.
Composers (%) | Difference between composers | |||||
---|---|---|---|---|---|---|
Bach | Beethoven | Chopin | Chi2 (df = 2) | p | ||
Recognition Codes | Style-C | 33.3 | 22.7 | 36.0 | 3.512 | > .1 |
Style-I | 14.7 | 18.7 | 12.0 | 1.317 | > .1 | |
Composer-C | 32.0 | 14.7 | 38.7 | 11.311 | < .003** | |
Composer-I | 17.3 | 29.3 | 36.0 | 6.724 | .035* | |
Content Codes | Pitch | 60.0 | 62.7 | 66.7 | 0.725 | > .1 |
Time | 80.0 | 77.3 | 66.7 | 3.947 | > .1 | |
Texture | 80.0 | 88.0 | 85.3 | 1.859 | > .1 | |
Type | 41.3 | 29.3 | 34.7 | 2.379 | > .1 | |
Experience | 48.0 | 46.7 | 66.7 | 7.545 | 0.023* |
Significance levels: * p < .05; *** p < .001.
Table 3 breaks down the percentages of recognition by actual composer. Here, as in the rest of the table, instances of the same code were only counted once per response (e.g., "Composer-I" indicates the presence of one or more incorrect responses of this kind). For Style recognition, both correct and incorrect responses were rather evenly divided between the composers (albeit with slightly lower Style recognition for Beethoven), but for recognition of Composer, Chi2-tests indicated larger differences between the three composers. Thus, while Composer-C was provided in about third of the trials for Bach and Chopin, the corresponding recognition rate for Beethoven was about half of this. As for the content codes, we see that statements regarding musical structure dominated the code strings: across the three composers, the majority of responses included information on Texture, Time, and Pitch. A significant difference in the content codes emerged only for Experience which received an elevated percentage in Chopin.
The Time of Recognition
As explained above, we hypothesized that correct style recognitions would take place in a rapid, intuitive manner, and that slower inferential processes might be triggered if such a rapid recognition is not forthcoming. In order to address this possibility, we compared the timing of the first correct and the first incorrect answers given in the verbal protocols. For each code type, Figure 2 shows the times of first occurrence from the flash of the score onscreen. It appears that the different codes, indeed, tended to appear at slightly different times. Thus, content concerning Texture and Time often occurred early on in the responses, while information about Type and Experience was typically given later (see Figure 2b).
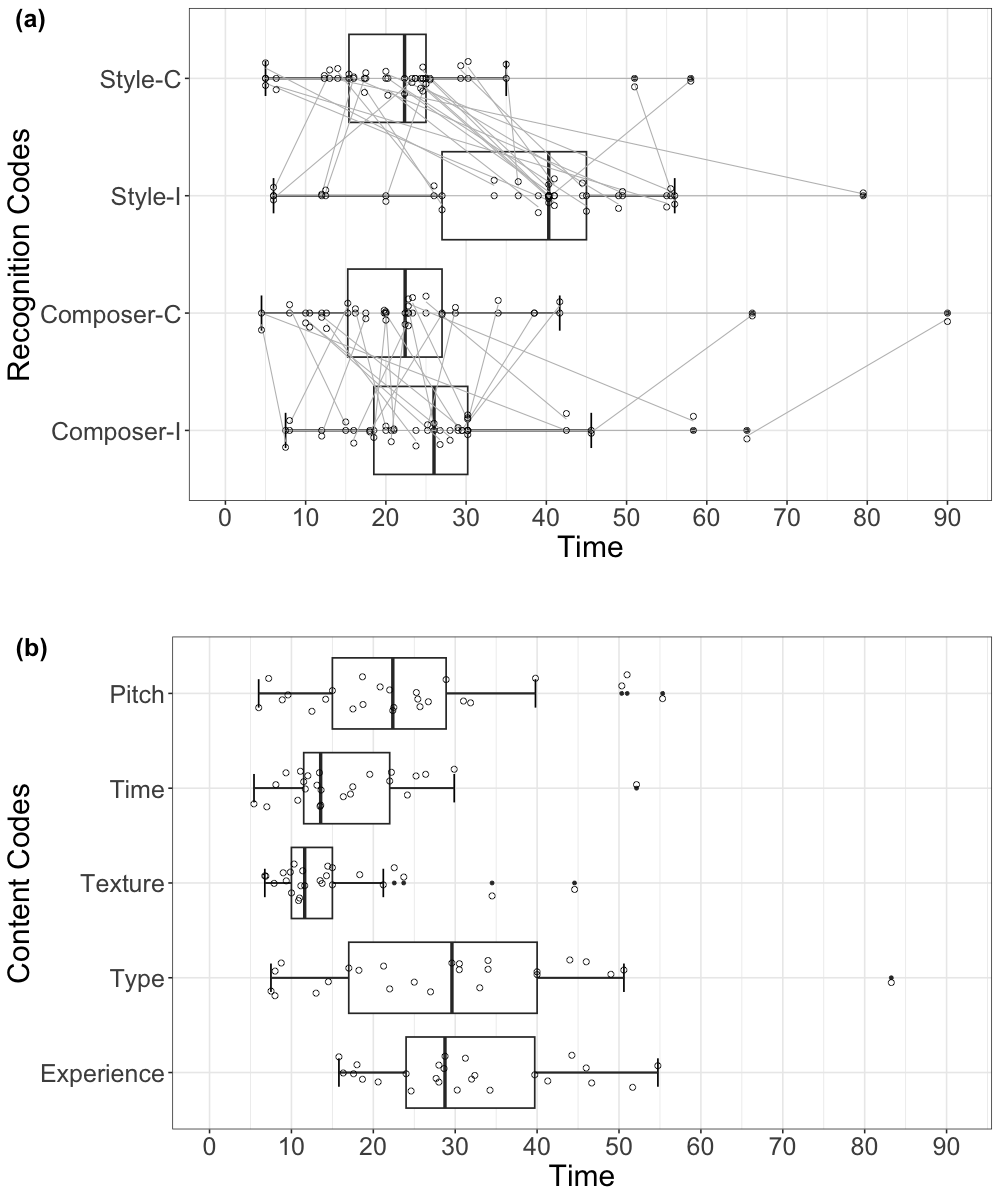
Figure 2. Participant means for the time of first code occurrence in verbal protocols, measured from the flash of the visual stimulus on the screen: (a) Recognition codes (with gray lines connecting individual participants "correct" and "incorrect" responses) and (b) Content codes. (See Table 2 for an explanation of the codes.)
For the recognition codes, the participant means in Figure 2a suggest some tendency for the correct recognitions to take place earlier than the incorrect ones. For a more formal statistical analysis, we used the original timing values (instead of means). The timings of the first occurrences of the four recognition codes were non-normally distributed (Shapiro-Wilk: all ps < .001, except for Style-I, p < .01). Thus, we analyzed the differences in the timing of the first correct and incorrect answers using the Wilcoxon rank-sum test for clustered data. Regarding Style, the test showed a significant difference between the timing of the first correct and incorrect responses available in the protocols (Z = –3.04, p = .002; n = 103, 24 clusters). The participants' grand mean for the first appearance of Style-C was 22.2 s after the flash of the stimulus (SD = 12.9) whereas the first Style-I codes occurred almost 15 s later, on average—at 36.7 s after the stimulus (SD = 19.1). No significant difference was found between the timing of Composer-C (grand mean = 25.6, SD = 19.8) and Composer-I (grand mean = 26.5, SD = 15.1; Z = –0.71, p = .546, n = 126, 25 clusters). In other words, correct recognitions did appear earlier than incorrect ones, but only in the case of generic statements concerning style period—not for statements regarding the assumed composer.
Richness of Spoken Content Preceding Recognition
In the introduction, we hypothesized that if correct recognitions result from an intuitive process, the responses indicating such recognition might also include less reflective content than in cases where the intuitive recognition fails. To address this issue, we carried out an analysis of "richness of content" in the verbal protocols, comparing the spoken contents preceding correct recognition and spoken contents when recognition did not take place. Richness of content was here operationalized as the number of different content codes occurring in recognition protocols before recognition (e.g., before the code Style-C), and in comparable stretches of the non-recognition protocols (where the aspect in question was not correctly recognized).
Our coding system had five different content codes (see Table 2), and thus the number of different types of content codes in a given response could vary between 0 and 5. In the case of recognition protocols, we took into account the number of different content codes preceding correct recognition (of Style or Composer). For a fair comparison with non-recognition protocols, we truncated each participant's non-recognition protocols at the average time of the first recognition codes in the same participant's recognition protocols, and considered only the content codes appearing before this point of time. For instance, participant Nr. 1 recognized Style-C in four trials, at the mean time of 20 s after the stimulus. For this participant, the remaining five trials lacking Style-C were truncated at 20 s, and only content codes occurring before this point of time were considered. A similar procedure was followed for determining appropriate amount of content to be considered in responses lacking Composer-C. In these analyses, we thus ignored all codes for incorrect recognition.
For comparing the richness of content between recognition and non-recognition protocols, we calculated the mean numbers of different content codes for each participant. Participants without any correct recognitions were removed from the respective analyses (two in the Style analysis, three in the Composer analysis). The remaining participants' mean numbers of different content codes are depicted in Figure 3. As the boxplots suggest, the number of different content codes was slightly higher in the non-recognition protocols than in the recognition protocols. According to paired t-tests, the difference was significant for Composer recognition (t(21) = –2.84, p < .01), but not for Style (t(22) = –1.60, p > .1; two-tailed tests). We may thus conclude that at least for Composer recognition, the participants tended to use more restricted descriptive contents before correct recognition than in comparable time periods when no correct recognition was forthcoming.
Total Spoken Content
Above, we saw that neither Composer-C nor Style-C recognition were supported by greater richness of spoken contents before the time of recognition. It remains to be seen whether such supportive content could have been available to the participants from the visual stimulus, even though only mentioned later in their protocols, after the recognition had already taken place. To check this option, we repeated the analysis regarding richness of content with all content codes available in the protocols (up to the first utterance of the interviewer). T-tests did not reveal significant differences in the numbers of different content codes, either between recognition and non-recognition of Style-C (t(22) = 1.13, p > .1) or between recognition and non-recognition of Composer-C (t(21) = –0.89, p > .1). Consequently, there was no evidence to support the idea that correct recognitions would have been accompanied by richer overall contents than were available when recognition did not take place.
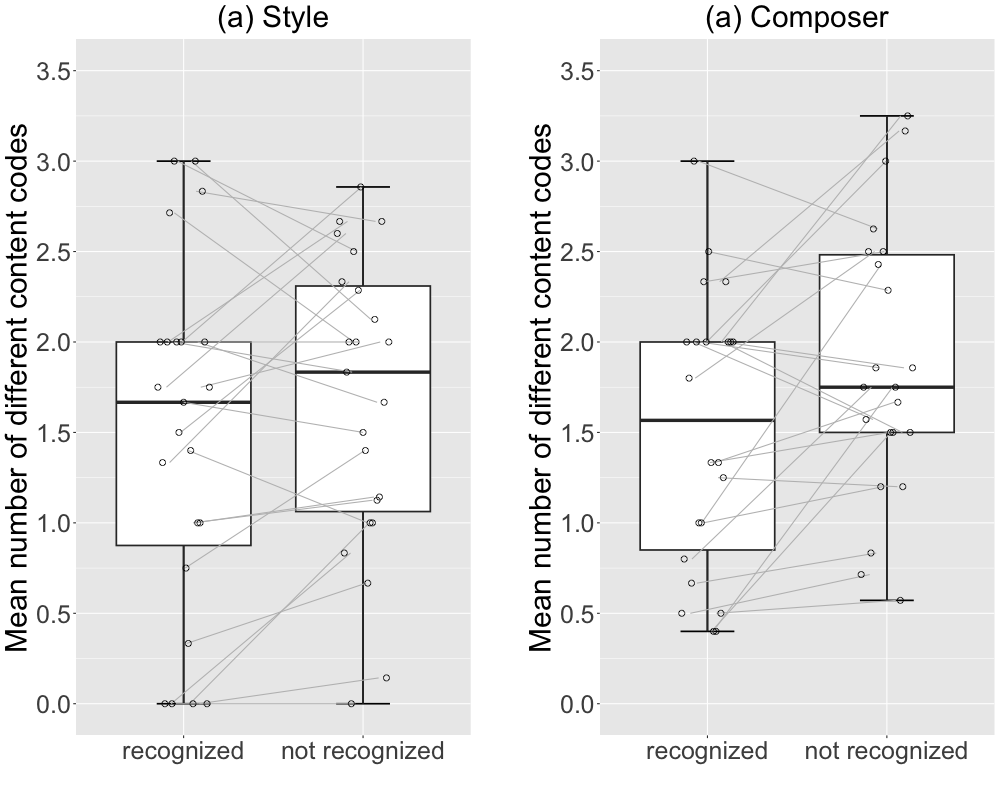
Figure 3. Richness of spoken content preceding correct recognition in the recognition protocols vs. in equally long stretches of the non-recognition protocols: (a) before recognition of Style, N = 23, and (b) before recognition of Composer, N = 22. Individual participants' means are connected with gray lines.
DISCUSSION
In this article, we studied classical pianists' visual recognition of musical style after a brief exposure to notated scores. Inspired by medical experts' rapid holistic processing of visual displays as well as research on music recognition based on reduced auditory information, we wanted to see whether expert classical pianists would be able to attribute appropriate style period to excerpts of written piano music after very brief exposure times. In the empirical study, 25 pianists were shown excerpts of piano scores by three composers, representing what are commonly known as the Baroque (J. S. Bach), Classical (early L. v. Beethoven), and Romantic periods (F. Chopin). After 500-ms exposure times, the participants described what they had seen, and attempted to assess the stylistic period in question. It turned out that recognition was, overall, very good: almost half of the verbal protocols included a correct assessment of either Style or Composer. Composer names were not called for by the task instructions, and thus it seems that they may have served as alternative basic-level categories in addressing musical style—providing an easy conceptual access to musical style, with an optimal balance between efficiency and informativeness (see Rosch et al., 1976). In pianists' verbal protocols, correct responses regarding both Style and Composer outnumbered the trials with corresponding incorrect responses, suggesting that the sizable recognition rates were not just due to excessive guessing.
Inspired by a dual-process account of cognition (Betsch, 2008; Betsch & Glöckner, 2010; Evans, 2010a; 2010b; Evans & Stanovich, 2013), we also wanted to find evidence for whether possible rapid style recognitions might take place by an intuitive, automatic process, or alternatively by a reflective, analytical process using selected musical features as inferential cues. For this purpose, we addressed the latency of the style attributions in the verbal protocols as well as the kinds of spoken contents preceding them. Regarding response latency, we found that correct responses regarding Style appeared significantly earlier in the spoken responses than incorrect ones. This is compatible with the notion of a default intuitive recognition process and a slower, reflective process of analysis when intuitive recognition is not forthcoming (Betsch & Glöckner, 2008; Evans, 2007; Evans & Stanovich, 2013). Regarding spoken contents, we found that before appropriate recognition of Composer, pianists mentioned fewer separate types of content than in comparable stretches of time when Composer was not recognized. Such a result would be compatible with intuitive recognition of Composer, as opposed to more analytical, inferential processes when such recognition failed.
The whole picture is not quite clear, however, given that the latency result was only obtained for the Style labels (but not for Composer names), and the content result was only found in the case of Composers (but not for Style). Nevertheless, our results do not support the alternative scenario in which appropriate recognitions of either Style or Composer would have been inferentially based on richer array of analytical information than was available in cases of non-recognition. To be sure, our interpretation in favor of intuitive thought processes is based on the idealized assumption that more inferential, reflective processes would have been directly attested in the spoken contents of the verbal protocols. However, given that participants typically started talking directly after the flash of the stimulus, we deem the protocols as relatively reliable indications of the reflective thought processes that took place after the exposure to the visual stimulus.
Akin to medical experts' rapid holistic grasp of radiographs (Sheridan & Reingold, 2017), professional pianists in our study were shown to derive relevant stylistic information from written piano music after very brief visual exposures. Decisions that would most certainly require analytical deliberation in early stages of musical learning were here made by experts by chiefly relying on intuitive processes (see Bangert et al., 2014). Quite often, the pianists did engage in inferential thought processes about what they had seen—even though the kinds of inference used would require another (qualitative) study. According to our present analysis, however, their stylistic recognitions appeared to take place despite such inferential thinking rather than because of it. The visual grasp of style seemed to function more like a pattern-recognition process than in terms of rational inference. Our results thus resonate with a host of popular accounts regarding the usefulness of experience-based intuition in professional contexts (e.g., Gladwell, 2005; Klein, 2003).
For some readers, the levels of recognition as such might seem like our most remarkable finding. For instance, the composer was recognized in 28% of all responses, and four of the participants recognized composers five times out of nine—not an insignificant feat after a 500-ms exposure to three systems of musical notation. There is an important caveat, though, that concerns the availability of suitable composer categories. We may recall the so-called availability heuristic in which subjective probabilities assigned to events may be increased by the ease with which relevant instances come to mind, causing systematic biases (Tversky & Kahneman, 1973). Here, a similar heuristic may have inflated the number of correct responses for both Style and Composer since the style periods and composers represented in our stimuli were all highly central to the history of Western art music. For instance, among the 148 instances of composer names in our data, 36 were given to composers usually classified within the Baroque period, but 29 of the latter (80.6%) were in favor of Bach, while Scarlatti received five responses and Rameau and Handel both only one. Similarly, Beethoven covered 50.0% of responses mentioning composers of the Classical period, and Chopin got 49.3% of responses given to composers from early to late Romanticism. Supposing that musical style periods are associated with various composite style patterns involving several musical parameters, musical excerpts representing less central composers of the same style periods might have received fewer correct responses—while Bach, for instance, might have continued to snatch most guesses in response to other composers' music with "Baroque" features. Future studies of brief exposure to musical scores should thus vary the representativeness of the composers within their respective style categories and consider the availability of relevant response labels by more carefully analyzing the musical knowledge of the participants.
The present results are also subject to other methodological caveats. Our main concern has not been in the relative ease of recognition of various musical styles, and thus researchers interested in such questions might find the present study wanting in some respects. First, the choice of Beethoven examples favored his eighteenth-century sonatas in a way that may explain the lower recognition percentages in these stimuli, in comparison to the other composers. Second, any study focusing more systematically on the various musical styles themselves should use a randomized stimulus order. Third, the differences in recognition might also be related with visual and/or musical complexity of the stimuli which was not controlled for in the current study. However, in a study addressing the recognition of different styles, any suggestions to use style-independent measures of complexity might also be called into question, given that the styles themselves may often differ in terms of the types of complexity involved.
Fourth, and most importantly, we were not able to control participants' prior familiarity with the musical works for fear that showing the scores after the session would allow some information to be leaked to other potential participants in the local pianist community. Even though the particular musical works were explicitly identified in relatively few of the verbal protocols (see above), these compositions as such could be expected to be relatively well-known among professional pianists. Therefore, our study does not allow a secure distinction between cases where a style (or composer) is recognized via general stylistic features typical of that style (or composer) and cases where a style (or composer) is recognized via veridical identification of specific pieces. To illustrate by an analogy with face recognition, these cases would correspond to the difference between recognizing "an elderly man" and recognizing "my father." Both kinds of recognition can be of interest for music reading, of course, but future studies should strive to tease them apart, for instance by involving stylistically appropriate musical stimuli generated by the use of artificial intelligence.
In this study, we have also not touched upon the question of how individual differences between experts might affect the processes of style recognition. In a previous study of classical pianists' silent memorizing of musical notation, it was found that successful recall of right-hand melodies was associated with higher aural skills, whereas left-hand recall was related to verbal cognitive style and analytical music-processing style (Loimusalo and Huovinen, 2018). Thus, while it may seem natural for musicians to insist on the primacy of the inner hearing of notated music, i.e., notational audiation (see Brodsky et al., 2003; 2008), some aspects of musical notation might require cognitive representations that are even better supported by other abilities and thinking habits. Quick recognition of musical style is an example of a notation-based task in which experts may excel even despite not having time for inner hearing of the notated music. Conceivably, high-level performance in this task might be supported by speed of information processing (see Kopiez & Lee, 2006; 2008) and a wide experientially-learned knowledge base regarding musical styles and their notational representations.
The skill of quick stylistic categorization may serve any user of musical notation from the music teacher to the musicologist. Emphatically, however, such recognition should not be seen as a cognitive trick that is separate from playing music. While most studies on sight-reading achievement operate on error rates (see Mishra, 2014), this may have the unfortunate tendency of reducing sight-reading skill to pressing the right keys at an approximately right time. Even supposing that the main challenge of reading music is just "to form adequate motor responses to perceived notation" (Fourie, 2004, p. 1), in the real world such achievements are also likely to involve the activation of stylistically appropriate action schemata for phrasing, micro-timing, and dynamics. Artistry in sight reading thus also depends on quick recognition of musical style. If the musician's span of "looking ahead" in the notation is around one or two seconds (see Furneaux & Land, 1999; Huovinen et al., 2018), this also dictates the limits for arriving at snap decisions about style-based interpretative changes required within a piece (e.g., between various topics in Mozart). In such contexts, it may be more musically convincing to jump into rough, fallible assumptions about stylistic character than to maintain a "neutral" interpretation until more information is secured. Our study encourages such leaps of faith: for experienced musicians, they can more often be right than wrong.
ACKNOWLEDGEMENTS
This article was copyedited by Matthew Moore and layout edited by Jonathan Tang.
NOTES
-
Correspondence can be addressed to: Erkki Huovinen, Royal College of Music in Stockholm, Box 27711, SE-11591 Stockholm, Sweden. E-mail: erkki.s.huovinen@gmail.com.
Return to Text
REFERENCES
- Bakdash, J. Z., & Marusich, L. R. (2017). Repeated measures correlation. Frontiers in Psychology, 8(456), 1–13. https://doi.org/10.3389/fpsyg.2017.00456
- Bakdash, J. Z., & Marusich, L. R. (2021). Package "rmcorr." R package version 0.4.3. https:// CRAN.R-project.org/package=rmcorr.
- Bangert, D., Schubert, E., & Fabian, D. (2014). A spiral model of musical decision-making. Frontiers in Psychology, 5(320), 1–11. https://doi.org/10.3389/fpsyg.2014.00320
- Bangert, D., Schubert, E., & Fabian, D. (2014). Practice thoughts and performance action: Observing processes of musical decision-making. Music Performance Research, 7, 27–46.
- Barrett, M. S. & Gromko, J. E. (2007). Provoking the muse: A case study of teaching and learning in music composition. Psychology of Music, 35, 213–230. https://doi.org/10.1177/0305735607070305
- Bayley, A. (2017). Cross-cultural collaborations with the Kronos Quartet. In E. F. Clarke & M. Doffman (Eds.), Distributed creativity: Collaboration and improvisation in contemporary music (pp. 93–113). New York: Oxford University Press. https://doi.org/10.1093/oso/9780199355914.003.0007
- Bean, K. L. (1938). An experimental approach to the reading of music. Psychological Monographs, 50(6), i–80. https://doi.org/10.1037/h0093540
- Belfi, A. M., Kasdan, A., Rowland, J., Vessel, E. A., Starr, G. G., & Poeppel, D. (2018). Rapid timing of musical aesthetic judgments. Journal of Experimental Psychology: General, 147, 1531–1543. https://doi.org/10.1037/xge0000474
- Betsch, T. (2008). The nature of intuition and its neglect in research on judgment and decision making. In H. Plessner, C. Betsch, & T. Betsch (eds.), Intuition in judgment and decision making (pp. 3–22). New York: Erlbaum. https://doi.org/10.4324/9780203838099
- Betsch, T. & Glöckner, A. (2010). Intuition in judgment and decision making: Extensive thinking without effort. Psychological Inquiry, 21, 279–294. https://doi.org/10.1080/1047840X.2010.517737
- Bigand, E., Filipic, S., & Lalitte, P. (2005). The time course of emotional responses to music. Annals of the New York Academy of Sciences, 1060, 429–437. https://doi.org/10.1196/annals.1360.036
- Brams, S., Ziv, G., Levin, O., Spitz, J., Wagemans, J., Williams, A. M., & Helsen, W. F. (2019). The relationship between gaze behavior, expertise, and performance: A systematic review. Psychological Bulletin, 145, 980–1027. https://doi.org/10.1037/bul0000207
- Brodsky, W., Henik, A., Rubinstein, B.-S., & Zorman, M. (2003). Auditory imagery from musical notation in expert musicians. Perception & Psychophysics, 65, 602–612. https://doi.org/10.3758/BF03194586
- Brodsky, W., Kessler, Y., Rubinstein, B.-S., Ginsborg, J., & Henik, A. (2008). The mental representation of music notation: Notational audiation. Journal of Experimental Psychology: Human Perception and Performance, 34, 427–445. https://doi.org/10.1037/0096-1523.34.2.427
- Burman, D. D. & Booth, J. R. (2009). Music rehearsal increases the perceptual span for notation. Music Perception, 26, 303–320. https://doi.org/10.1525/mp.2009.26.4.303
- Chase, W. G. & Simon, H. A. (1973). Perception in chess. Cognitive Psychology, 4, 55–81. https://doi.org/10.1016/0010-0285(73)90004-2
- Deutsch, D. (1980). The processing of structured and unstructured tonal sequences. Perception & Psychophysics, 28, 381–389. https://doi.org/10.3758/BF03204881
- Drew, T., Evans, K., Võ, M. L.-H., Jacobson, F. L., & Wolfe, J. M. (2013). Informatics in radiology: What can you see in a single glance and how might this guide visual search in medical images? RadioGraphics, 33, 263–274. https://doi.org/10.1148/rg.331125023
- Ericsson, K. A. & Simon, H. A. (1993). Protocol analysis: Verbal reports as data. Revised ed. Cambridge, Mass. & London: The MIT Press. https://doi.org/10.7551/mitpress/5657.001.0001
- Evans, J. St. B. T. (2007). On the resolution of conflict in dual process theories of reasoning. Thinking & Reasoning, 13, 321–339. https://doi.org/10.1080/13546780601008825
- Evans, J. St. B. T. (2010a). Thinking twice: Two minds in one brain. Oxford: Oxford University Press.
- Evans, J. St. B. T. (2010b). Intuition and reasoning: A dual-process perspective. Psychological Inquiry, 21, 313–326. https://doi.org/10.1080/1047840X.2010.521057
- Evans, J. St. B. T. & Stanovich, K. E. (2013). Dual-process theories of higher cognition: Advancing the debate. Perspectives on Psychological Science, 8, 223–241. https://doi.org/10.1177/1745691612460685
- Falthin, A. (2015). Meningserbjudanden och val: En studie om musicerande i musikundervisning på högstadiet. ["Affordance and choice: Performing music in lower secondary school."] Stockholm: Royal College of Music in Stockholm and Lund University.
- Fine, P. A., Wise, K. J., Goldemberg, R., & Bravo, A. (2015). Performing musicians' understanding of the terms "mental practice" and "score analysis." Psychomusicology: Music, Mind, and Brain, 25, 69–82. https://doi.org/10.1037/pmu0000068
- Fourie, E. (2004). The processing of music notation: Some implications for piano sight-reading. Journal of the Musical Arts in Africa, 1, 1–23. https://doi.org/10.2989/18121000409486685
- Fournier, G., Moreno Sala, M. T., Dubé, F., & O'Neill, S. (2019). Cognitive strategies in sight-singing: The development of an inventory for aural skills pedagogy. Psychology of Music, 47, 270–283. https://doi.org/10.1177/0305735617745149
- Furneaux, S., & Land, M. F. (1999). The effects of skill on the eye-hand span during musical sight-reading. Proceedings of the Royal Society of London, Series B, 266(1436), 2435–2440. https://doi.org/10.1098/rspb.1999.0943
- Gilman, E. & Underwood, G. (2003). Restricting the field of view to investigate the perceptual spans of pianists. Visual Cognition, 10, 201–232. https://doi.org/10.1080/713756679
- Gjerdingen, R. O. & Perrott, D. (2008). Scanning the dial: The rapid recognition of music genres. Journal of New Music Research, 37, 93–100. https://doi.org/10.1080/09298210802479268
- Gladwell, M. (2005). Blink: The power of thinking without thinking. New York: Little, Brown and Company.
- Glaser, B. G. & Strauss, A. L. (1967). The discovery of grounded theory: Strategies for qualitative research. Hawthorne, N.Y.: Aldine de Gruyter. https://doi.org/10.1097/00006199-196807000-00014
- Gobet, F. & Simon, H. A. (2000). Five seconds of sixty? Presentation time in expert memory. Cognitive Science, 24, 651–682. https://doi.org/10.1207/s15516709cog2404_4
- Gobet, F. (2020). The classic expertise approach and its evolution. In P. Ward, J. M. Schraagen, J. Gore, & E. Roth (Eds.), The Oxford handbook of expertise (pp. 35–55). New York: Oxford University Press. https://doi.org/10.1093/oxfordhb/9780198795872.013.2
- Goolsby, T. W. (1994a). Eye movement in music reading: Effects of reading ability, notational complexity and encounters. Music Perception, 12, 77–96. https://doi.org/10.2307/40285756
- Goolsby, T. W. (1994b). Profiles of processing. Eye movements during sightreading. Music Perception, 12, 96–123. https://doi.org/10.2307/40285757
- Grill-Spector, K. & Kanwisher, N. (2005). Visual recognition: As soon as you know it is there, you know what it is. Psychological Science, 16, 152–160. https://doi.org/10.1111/j.0956-7976.2005.00796.x
- de Groot, A. (1965). Thought and choice in chess. The Hague & Paris: Mouton & Co.
- Halpern, A. R. & Bower, G. H. (1982). Musical expertise and melodic structure in memory for musical notation. American Journal of Psychology, 95, 31–50. https://doi.org/10.2307/1422658
- Hultberg, C. (2002). Approaches to music notation: The printed score as a mediator of meaning in Western tonal tradition. Music Education Research, 4, 185–197. https://doi.org/10.1080/1461380022000011902
- Huovinen, E., Ylitalo, A.-K., & Puurtinen, M. (2018). Early attraction in temporally controlled sight reading of music. Journal of Eye Movement Research, 11(2):3, 1–30. https://doi.org/10.16910/jemr.11.2.3
- Hurme, T.-R., Puurtinen, M., & Gruber, H. (2019). When "doing" matters: The emergence of group-level regulation in planning for a music lesson. Music Education Research, 21, 52–70. https://doi.org/10.1080/14613808.2018.1484435
- Jiang, Y., He, X., Lee, M.-L. T., Rosner, B., & Yan, J. (2020). Wilcoxon rank-based tests for clustered data with R package clusrank. Journal of Statistical Software, 96(6), 1–26. https://doi.org/10.18637/jss.v096.i06
- Kalakoski, V. (2007). Effect of skill level on recall of visually presented patterns of musical notes. Scandinavian Journal of Psychology, 48, 87–96. https://doi.org/10.1111/j.1467-9450.2007.00535.x
- Kim, Y. J., Song, M. K., Atkins, R. (2020). What is your thought process during sight-reading? Advanced sight-reader's strategies across different tonal environments. Psychology of Music 00(0), 1–18.
- Klein, G. (2003). The power of intuition: How to use your gut feelings to make better decisions at work. New York: Currency Doubleday.
- Kopiez, R. & Lee, J. I. (2006). Towards a dynamic model of skills involved in sight reading music. Music Education Research, 8, 97–120. https://doi.org/10.1080/14613800600570785
- Kopiez, R. & Lee, J. I. (2008). Towards a general model of skills involved in sight reading music. Music Education Research, 10, 41–62. https://doi.org/10.1080/14613800701871363
- Krumhansl, C. L. (2010). Plink: "Thin slices" of music. Music Perception, 27, 337–354. https://doi.org/10.1525/mp.2010.27.5.337
- Kundel, H. L. & Nodine, C. F. (1975). Interpreting chest radiographs without visual search. Radiology, 116, 527–532. https://doi.org/10.1148/116.3.527
- Kundel, H. L., Nodine, C. F., Conant, E. F., & Weinstein, S. P. (2007). Holistic Component of Image Perception in Mammogram Interpretation: Gaze-tracking Study. Radiology, 242, 396–402. https://doi.org/10.1148/radiol.2422051997
- Lim, Y., Park, J. M., Rhyu, S.-Y., Chung, C. K., Kim, Y, & Yi, S. W. (2019). Eye-hand span is not an indicator of but a strategy for proficient sight-reading in piano performance. Scientific Reports, 9(17906), 1–11. https://doi.org/10.1038/s41598-019-54364-y
- Loimusalo, N. & Huovinen, E. (2018). Memorizing silently to perform tonal and nontonal notated music: A mixed-methods study with pianists. Psychomusicology: Music, Mind, and Brain, 28, 222–239. https://doi.org/10.1037/pmu0000227
- Loimusalo, N. & Huovinen, E. (2021). Expert pianists' practice perspectives: A production and listening study. Musicae Scientiae, 25, 480–508. https://doi.org/10.1177/1029864920938838
- Love, K. G. & Barrett, M. S. (2016). A case study of teaching and learning strategies in an orchestral composition masterclass. Psychology of Music, 44, 830–846. https://doi.org/10.1177/0305735615594490
- Mace, S. T., Wagoner, C. L., Teachout, D. J., & Hodges, D. A. (2011). Genre identification of very brief musical excerpts. Psychology of Music, 40, 112–128. https://doi.org/10.1177/0305735610391347
- Martinez, L., Falvello, V. B., Aviezer, H., & Todorov, A. (2016). Contributions of facial expressions and body language to the rapid perception of dynamic emotions. Cognition and Emotion, 30, 939–952. https://doi.org/10.1080/02699931.2015.1035229
- McPherson, G. E. (1994). Factors and abilities influencing sightreading skill in music. Journal of Research in Music Education, 42, 217–231. https://doi.org/10.2307/3345701
- McPherson, G. E. (2005). From child to musician: Skill development during the beginning stages of learning an instrument. Psychology of Music, 33, 5–35. https://doi.org/10.1177/0305735605048012
- McPherson, G. E. & Gabrielsson, A. (2002). From sound to sign. In R. Parncutt & G. E. McPherson (Eds.), The science and psychology of music performance: Creative strategies for teaching and learning (pp. 99–115). New York: Oxford University Press. https://doi.org/10.1093/acprof:oso/9780195138108.003.0007
- Meyer, L. B. (1989). Style and music: Theory, history, and ideology. Philadelphia: University of Pennsylvania Press.
- Mishra, J. (2014). Improving sightreading accuracy: A meta-analysis. Psychology of Music, 42, 131–156. https://doi.org/10.1177/0305735612463770
- Nodine, C. F. & Kundel, H. L. (1987). The cognitive side of visual search in radiology. In J. K. O'Regan and A. Levy-Schoen (Eds.), Eye Movements: From Physiology to Cognition (pp. 573–582). Elsevier. https://doi.org/10.1016/B978-0-444-70113-8.50081-3
- Ortmann, O. (1934). Elements of chord-reading in music notation. The Journal of Experimental Education, 3, 50–57. https://doi.org/10.1080/00220973.1934.11009965
- Penttinen, M., Huovinen, E., & Ylitalo, A.-K. (2013). Silent music reading: Amateur musicians' visual processing and descriptive skill. Musicae Scientiae, 17, 198–216. https://doi.org/10.1177/1029864912474288
- Peretz, I., Gagnon, L., & Bouchard, B. (1998). Music and emotion: Perceptual determinants, immediacy, and isolation after brain damage. Cognition, 68, 111–141. https://doi.org/10.1016/S0010-0277(98)00043-2
- Petitmengin, C. (2006). Describing one's subjective experience in the second person: An interview method for the science of consciousness. Phenomenology and the Cognitive Sciences, 5, 229–269. https://doi.org/10.1007/s11097-006-9022-2
- Rayner, K. (2009). The 35th Sir Frederick Bartlett lecture: Eye movements and attention in reading, scene perception, and visual search.. The Quarterly Journal of Experimental Psychology, 62, 1457–1506. https://doi.org/10.1080/17470210902816461
- R Core Team (2019). R: A language and environment for statistical computing. R Foundation for Statistical Computing, Vienna. https://www.R-project.org/.
- Reingold, E. M., Charness, N., Pomplun, M., & Stampe, D. M. (2001). Visual span in expert chess players: Evidence from eye movements. Psychological Science, 12, 48–55. https://doi.org/10.1111/1467-9280.00309
- Rosch, E., Mervis, C. B., Gray, W. D., Johnson, D. M., & Boyes-Braem, P. (1976). Basic objects in natural categories. Cognitive Psychology, 8, 382–439. https://doi.org/10.1016/0010-0285(76)90013-X
- Rosner, B., Glynn, R. J., Lee, M.-L. T. (2003). Incorporation of clustering effects for the Wilcoxon rank sum test: A large-sample approach. Biometrics, 59, 1089–1098. https://doi.org/10.1111/j.0006-341X.2003.00125.x
- Rule, N. O. & Ambady, N. (2008). Brief exposures: Male sexual orientation is accurately perceived at 50 ms. Journal of Experimental Social Psychology, 44, 1100–1105. https://doi.org/10.1016/j.jesp.2007.12.001
- Sheridan, H. & Reingold, E. M. (2017). The holistic processing account of visual expertise in medical image perception: A review. Frontiers in Psychology, 8(1620), 1–11. https://doi.org/10.3389/fpsyg.2017.01620
- Sloboda, J. A. (1976). Visual perception of musical notation: Registering pitch symbols in memory. Quarterly Journal of Experimental Psychology, 28, 1–16. https://doi.org/10.1080/14640747608400532
- Sloboda, J. A. (1982). Music performance. In D. Deutsch (Ed.), The psychology of music (pp. 479–496). New York: Academic Press. https://doi.org/10.1016/B978-0-12-213562-0.50020-6
- Truitt, F. E., Clifton, C., Pollatsek, A., & Rayner, K. (1997). The perceptual span and the eye-hand span in sight reading music. Visual Cognition, 4, 143–161. https://doi.org/10.1080/713756756
- Tversky, A. & Kahneman, D. (1973). Availability: A heuristic for judging frequency and probability. Cognitive Psychology, 5, 207–232. https://doi.org/10.1016/0010-0285(73)90033-9
- Virtanen, M. (2007). Musical works in the making: Verbal and gestural negotiation in rehearsals and performances of Einojuhani Rautavaara's piano concerti. Turku: University of Turku.
- Waters, A. J., Townsend, E., & Underwood, G. (1998). Expertise in musical sight reading: A study of pianists. British Journal of Psychology, 89, 123–149. https://doi.org/10.1111/j.2044-8295.1998.tb02676.x
- Waters, A. J., Underwood, G., & Findlay, J. M. (1997). Studying expertise in music reading: Use of a pattern-matching paradigm. Perception & Psychophysics, 59, 477–488. https://doi.org/10.3758/BF03211857
- Willis, J. & Todorov, A. (2006). First impressions: Making up your mind after a 100-ms exposure to a face. Psychological Science, 17, 592–598. https://doi.org/10.1111/j.1467-9280.2006.01750.x
- Wong, Y. K. & Gauthier, I. (2010). Holistic processing of musical notation: Dissociating failures of selective attention in experts and novices. Cognitive, Affective, & Behavioral Neuroscience, 10, 541–551. https://doi.org/10.3758/CABN.10.4.541
- Wong, Y. K., Lui, K. F. H., & Wong, A. C.-N. (2020). A reliable and valid tool for measuring visual recognition ability with musical notation. Behavior Research Methods, 53, 836–845. https://doi.org/10.3758/s13428-020-01461-w
- Zhang, J. & Mueller, S. T. (2005). A note on ROC analysis and non-parametric estimate of sensitivity. Psychometrika, 70, 203–212. https://doi.org/10.1007/s11336-003-1119-8